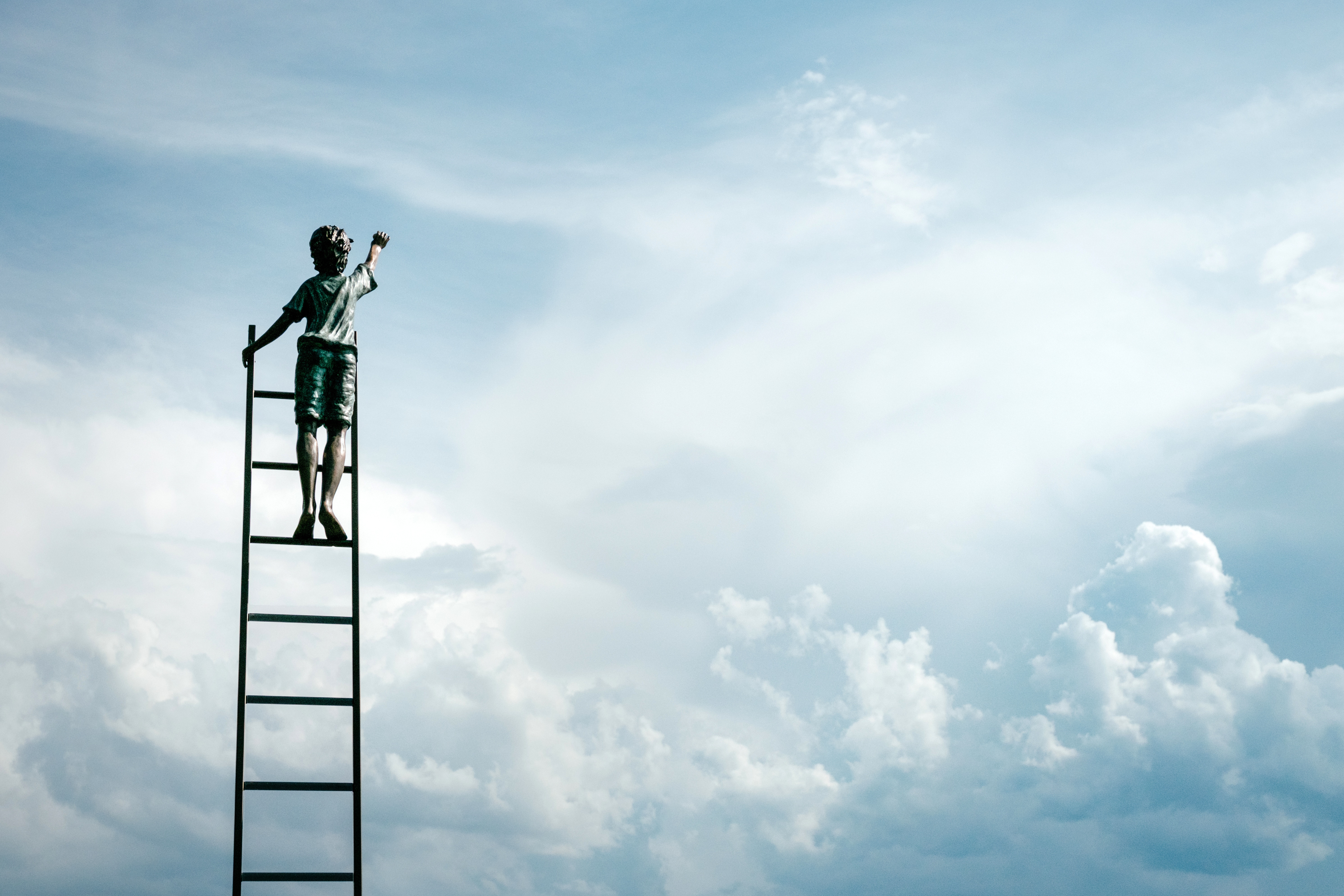
The BETTER program presents a number of challenges for state-of-the-art language technology. First, BETTER is concerned with fine-grained events and entities. Current distributed representations of text rely on shallow co-occurrence signals and one-size-fits-all similarity metrics which fail to capture the subtle semantic distinctions that are likely to be important to the program goals. Second, BETTER requires systems to be customized given extremely limited training data, in some cases harnessing only a handful of labels in order to adapt the system to a user's specific needs. Current state-of-the-art systems typically require hundreds of thousands of training examples in order to reach a practical level of performance. Third, BETTER requires that the systems produce seamless cross-lingual adaptation, maintaining top-level performance despite being applied to a language other than that on which the system was trained.
Our team at Brown University, the University of Pennsylvania and Ohio State University was awarded an IARPA BETTER grant entitled "Task and User-Aware Representation Learning for Fine-Grained Cross-Lingual Information Retrieval".