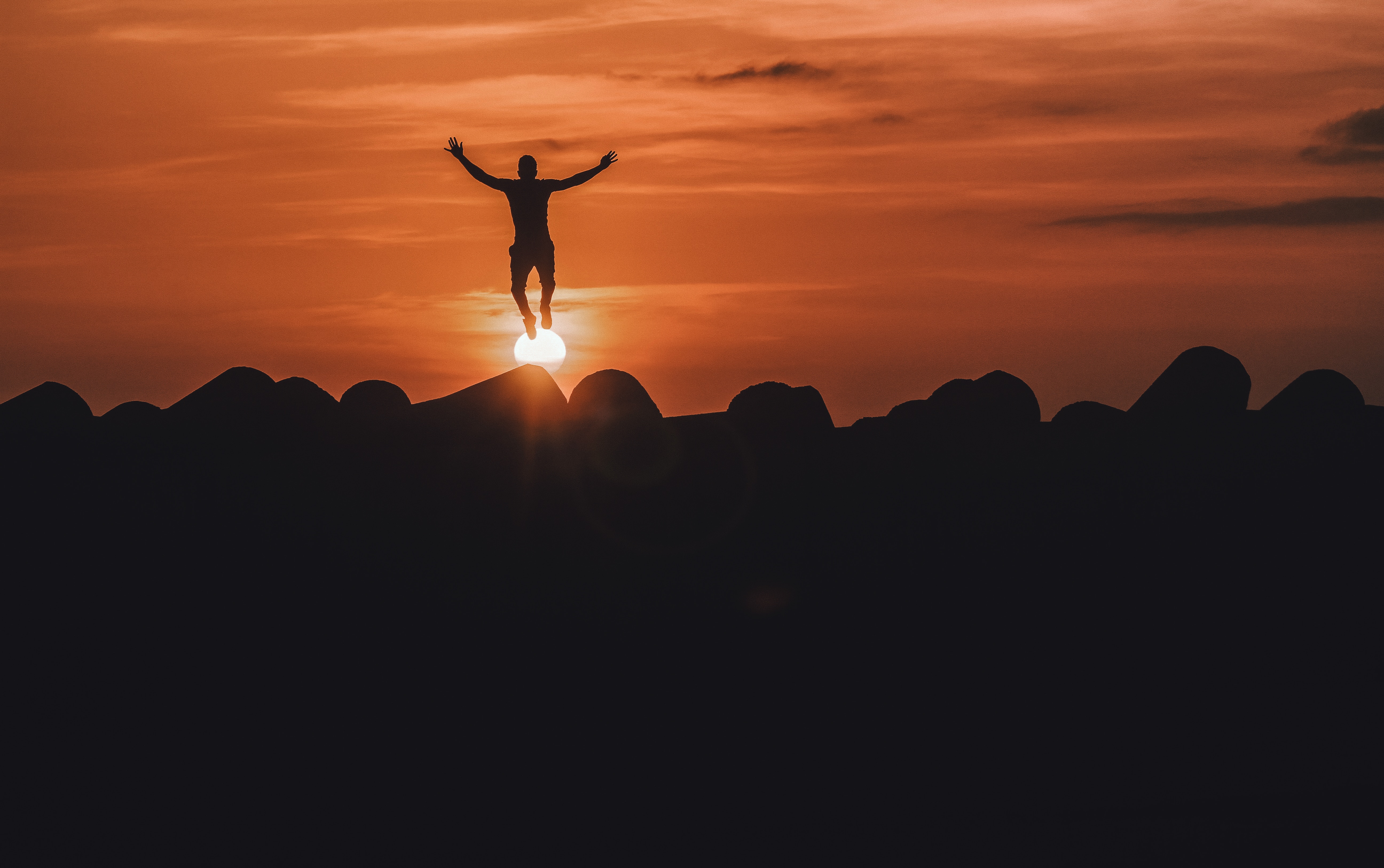
The past few decades have seen explosive growth in development and training of AI systems, which are now embodied in digital computing processes spanning several key industries. One area that has benefited from AI, and specifically Machine Learning (ML) techniques and statistical methods, is the area of Human Language Technology (HLT). However, ML suffers from a need of large amounts of annotated data for training in order to achieve the required accuracy for the various applications. ML technology is also brittle, incapable of dealing with new data sources, topics, media, and vocabularies. These weaknesses of ML as applied to natural language are due to exclusive reliance on the statistical aspects of language, with no regard for its meaning.
The Grounded Artificial Intelligence Language Acquisition (GAILA) project will focus on enabling computers to acquire language in a manner similar to the way children do. The process of observing moving images and aligning them with auditory stimuli allows them to associate a sequence of sounds (a word) with some aspect of the concrete or abstract elements of the world that the word represents.
Our team at Brown University was awarded a DARPA GAILA grant entitled "Cognitively Motivated Word Learning in Embodied Virtual Agents".