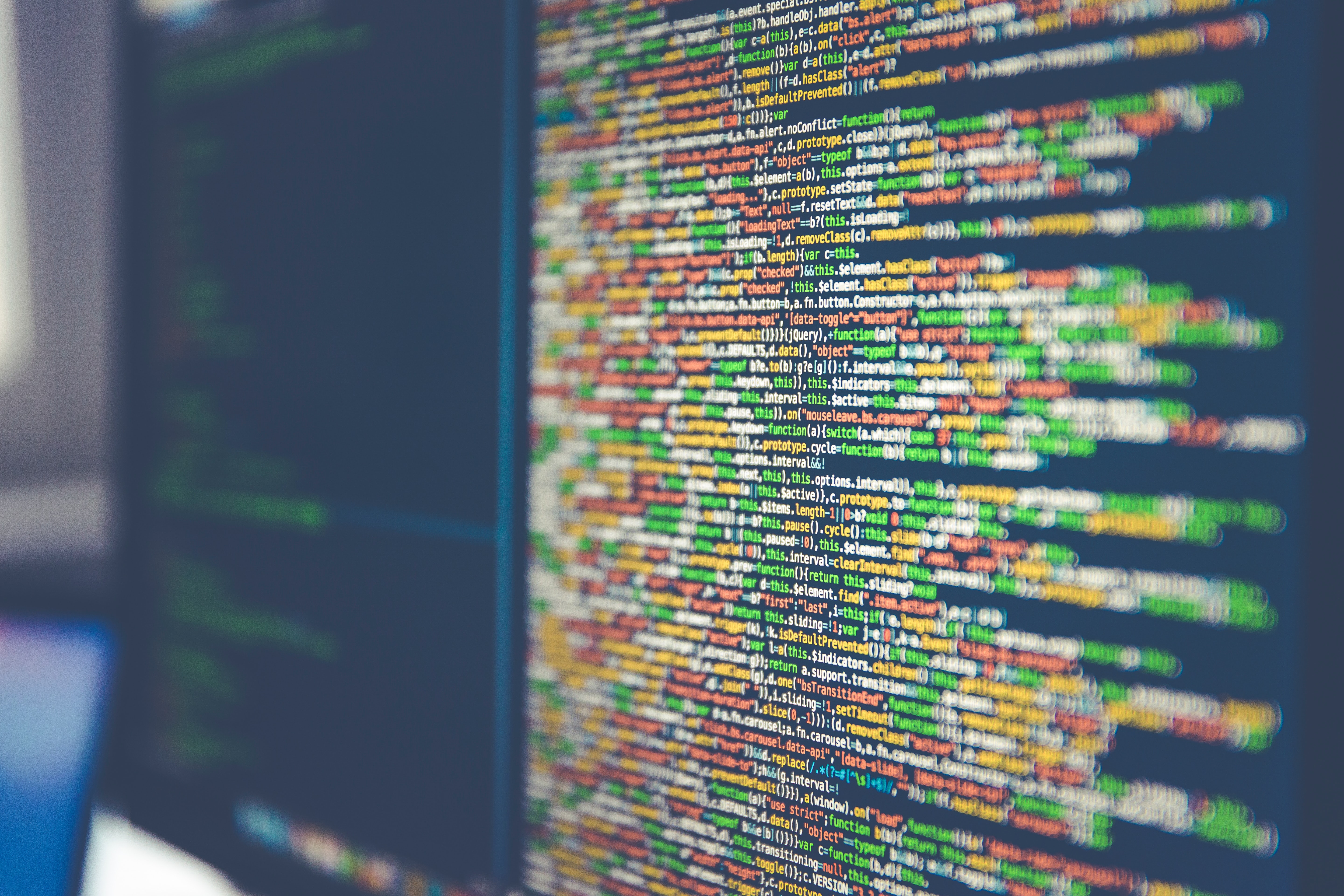
Search engines have become modern society's main sources of information. They put vast amounts of knowledge about virtually any topic at our fingertips wherever we go. To do so, a search engine studies our search query and tries to form an understanding of what it is that we were looking for in the first place, when querying for "boston tea party reason", "IRS form 1040" or "pizza near me". This internal representation is then compared with billions of webpages, books, or news articles in an attempt to find the best possible information for our searcher's query. This NSF Medium project will support and innovate this process in two ways. First, it will develop a more accurate understanding of search queries using insights into the way that humans use language, rather than just comparing queries and documents word-by-word. Secondly, using these improved representations of query meaning, the researchers will develop a fundamentally different way of searching for information. Instead of comparing our query with every possible match, they let the search engine come up with an idealized response to the query and then try to find those webpages that are most similar to this optimal answer. The expected consequences will be better search results and faster computation for the machines running the search engines (that, in turn, can lead to reduced electricity demand and CO2 emissions).
The lab was awarded an NSF Medium grant entitled "Generative Neural Information Retrieval Models" with start date September 1st. All forthcoming project updates, publications and deliverables will be shared on the project website https://osf.io/ar9gq/