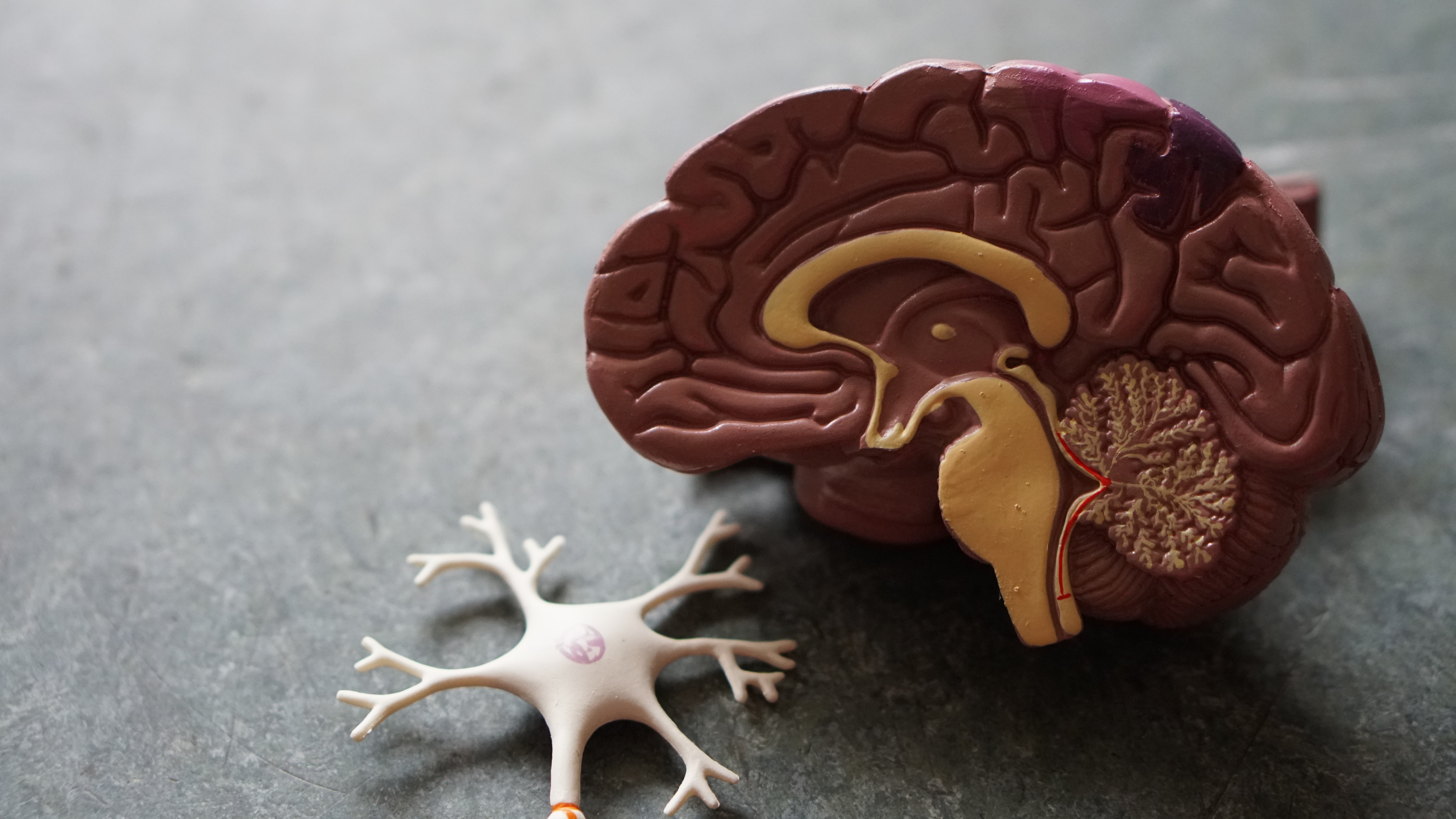
Strokes are acute medical conditions in which poor blood flow to a part of the brain results in neuronal death. Worldwide, stroke is the second most common cause of death, and the leading cause of physical disability. There are long-term risks associated with stroke: Inability to move, or feel, one side of the body, problems in verbal expression, or loss of vision, among others. Two lines of action must be taken in order to minimize these long-term risks. Firstly, an urgent intervention in the first hours after the stroke is critical. Secondly, the continuous monitorization during the rehabilitation phase is also critical, to ensure a positive outcome and minimize risks. According to the same SAFE report, only 30% of the survivors receive unit care, and despite its importance, access to rehabilitation and long-term support is a known problem.
Augusto Garcia-Agundez has been awarded an ERC Marie Skłodowska-Curie Fellowship to explore wearable sensors and deep learning technologies to improve the effectiveness of monitorization during the rehabilitation phase after stroke. Among others, Deep Learning with Convolutional Neural Networks (CNN), Recurrent Neural Networks (RNN), Generative Adversarial Networks (GAN), Natural Language Processing (NLP), Outlier Detection (OD) and Distributed Parallel Computing (DPC) techniques will be used to improve the monitorization of rehabilitation effectiveness and adherence, and determine the predicted outcome of the patient after the rehabilitation process.