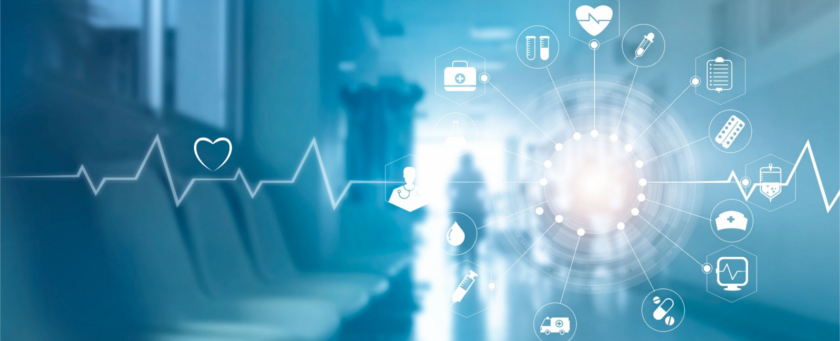
When developing clinical treatment plans for patients, especially for difficult or previously unseen conditions, medical practitioners often rely on experience made with comparable cases either from their own past experience or described in the scientific literature. An important goal in medical informatics is supporting decision making by identifying the literature, the patients that have similar characteristics and the procedures, treatments or laboratory tests that are relevant in the context of a specific patient. In spite of the growing volume of available medical journals and digitized patient records, this often turns out to be a challenging task. Finding reference cases requires methods to summarize, model and search large-scale collections of heterogeneous medical information. This data is typically highly diverse and multi-modal, including physiological measurements, drug prescriptions, procedures, unstructured clinical notes (text) and to an increasing extent, also genetic information.
Carsten received a four-year NSF Ambizione Career Award to develop deep machine learning technologies to summarize and interpret this data, empowering medical experts to extract meaning from big medical datasets and biomedical publications.